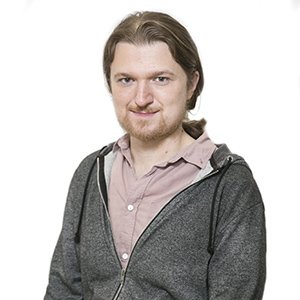
ICREA Research Professor, ERC Starting Grant

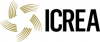
Meet Our Scientists Videos
Research information
Background
Genomic, transcriptomic and epigenomic ‘big data’ presents opportunities to learn about the properties of living systems and to treat diseases more successfully. In order to address outstanding questions in biology and biomedicine, we use statistical genome analyses and machine learning methods to discover robust and biologically meaningful patterns. We study omics data originating from human tumours (somatic mutations, transcriptomes), human populations (germline variation, epigenomes) and metagenomic data (including the human microbiome).
Research interests
In the GenomeDataLab, we study the mechanisms underlying maintenance of genome integrity mechanisms of maintaining genome integrity in human cells via statistical analyses of mutation patterns in cancer. Next, we are interested in how mRNA synthesis and turnover pathways shape genomes and transcriptomes in health and disease. Finally, we combine experimental work and genomics to scan cancer genomes for driver genes and for genetic interactions to predict tumour evolution and identify novel synthetic lethalities. For more information, please see https://www.genomedatalab.org/research-interests
Research lines
- Genomics of DNA repair. We aim to understand mechanisms of maintaining genome integrity in human cells via statistical analyses of mutation patterns in cancer. Read more >>>
- mRNA quality control. We are interested in how the pathways dealing with synthesis and turnover of mRNA shape genomes and transcriptomes, in health and disease. Read more >>>
- Predicting cancer evolution. Combining experiment and genomics, we scan cancer genomes for causal genes and for genetic interactions to better understand tumor evolution. Read more >>>
- Bioinformatics of gene function. From E. coli to human, the function of a substantial fraction of their genes is unknown; we think that machine learning approaches can help solve this. Read more >>>
Selected publications
Projects
"Mutabilidad regional del genoma en células somáticas humanas" (RegioMut), cofinanciado por el Ministerio de Ciencia, Innovación y Universidades- Agencia Estatal de Investigacióny por el Fondo Europeo de Desarrollo Regional (FEDER) de la Unión Europea. Referencia: BFU2017-89833-P.
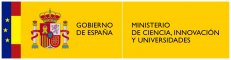


"Hypermutated tumors: insight into genome maintenance and cancer vulnerabilities provided by an extreme burden of somatic mutations" (HYPER-INSIGH), financiado por el European Research Council (ERC) mediante el Programa de Investigación e Innovación de la Unión Europea Horizonte 2020. Referencia: 757700.
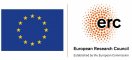
Grup de Recerca consolidat (SGR 2017-2019) de la Secretaria d'Universitats i Recerca del Departament d'Empresa i Coneixement de la Generalitat de Catalunya. Agencia de Gestió d'Ajuts Universitaris i de Recerca (AGAUR). Referencia: 2017 SGR 1322.

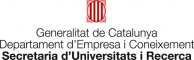
"Improved clinical decisions via integrating multiple data levels to overcome chemotherapy resistance in high-grade serous ovarian cancer" (DECIDER), financiado por la Comisión Europea mediante el Programa de Investigación e Innovación de la Unión Europea Horizonte 2020. Referencia: 965193.

EMBO Young Investigator Programme Award, 2020.
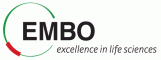
Suport obtingut de l’FSE a través de les Ajuts per a la contractació de personal investigador novell (FI). El Fons Social Europeu dona suport a la creació d’ocupació, ajuda a las persones a aconseguir millors llocs de treball i garanteix oportunitats laborals més justes per a la ciutadania de la Unió Europea.
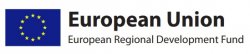
“The genomic landscape of mutagenesis resulting from DNA repair failures in human tumors” (REPAIRSCAPE) funded by the Spanish Research State Agency (PID2020-118795GB-I00/ AEI /10.13039/501100011033).

Grup de Recerca consolidat (SGR-Cat 2021) del Departament de Recerca i Universitats. Agència de Gestió d'Ajuts Universitaris i de Recerca (AGAUR). Referència: 2021 SGR 00616

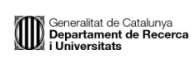
"Risk Factors Toolbox, lung cancer, prevention, screening, health cohorts, wearables, sensors" (LUCIA), financiado por la Comisión Europea mediante el Programa de Innovación e Investigación de la Unión Europea Horizonte Europa. Referencia: 101096473

"Potentiating cancer immunotherapy by inhibiting the NMD quality-control pathway guided by genetic markers" (POTENT-IMMUNO), financiado por Fundación "la Caixa" mediante la convocatoria CaixaResearch Health 2022. Referencia: HR22-00402
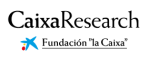